GUSTAVO PÉREZ
Postdoctoral Scholar at UC Berkeley (EECS)
COMPUTER VISION & MACHINE LEARNING—IMAGE UNDERSTANDING | AI & HUMAN-IN-THE-LOOP | AI FOR SCIENCE
ABOUT ME
I am a Postdoctoral Scholar at UC Berkeley EECS working with Stella Yu and Miki Lustig. I got my Ph.D. at UMass Amherst CICS where I worked with Subhransu Maji in the Computer Vision Lab. Previously, I spent three years as a graduate research assistant working with Pablo Arbeláez in the Biomedical Computer Vision Group at the Universidad de Los Andes, where I got my Master's degree in Biomedical Engineering. I got my Bachelor's degree in Electronics Engineering from the Universidad del Norte in Barranquilla, Colombia.
From data to science with AI and human-in-the-loop→go to research
My research in computer vision and machine learning focuses on finding effective ways to combine human and computational effort to facilitate accurate scientific measurement in large datasets. Additionally, my research focuses on optimal learning of deep learning models.
My work is also interdisciplinary, where I collaborate with ecologists to study phenology and population declines on bird species using RADAR imagery→(project page), with astronomers to provide insights into the process of star formation and the birth and evolution of galaxies from the Hubble Space Telescope (HST) and the James Webb Space Telescope (JWST) data→(project page), and with chemists to predict absorption of nanoporous materials→(project page). My research goal is to create data-efficient solutions to enable fundamental scientific advances.
Here is my research statement, CV, and a list with my publications.
SELECTED RESEARCH
DISCOUNT—Counting in Large Image CollectionsWe contribute counting methods for large image collections where images are freely available and it is possible to train a detector to run on all images, but the detector is not reliable enough for the final counting task, or its reliability is unknown.Github code repository · paper · press release |
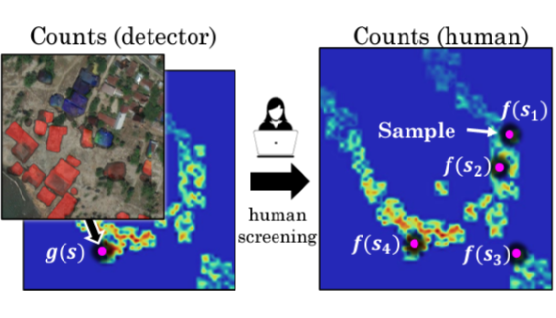
AI FOR ECOLOGY—Insectivore Response to Environmental ChangeRoost detection from weather radar data to investigate the behavior of three aerial insectivore species as bellwethers for environmental change and ecosystem health: Purple Martin, Tree Swallow, and Mexican free-tailed BatGithub code repository · preprint |
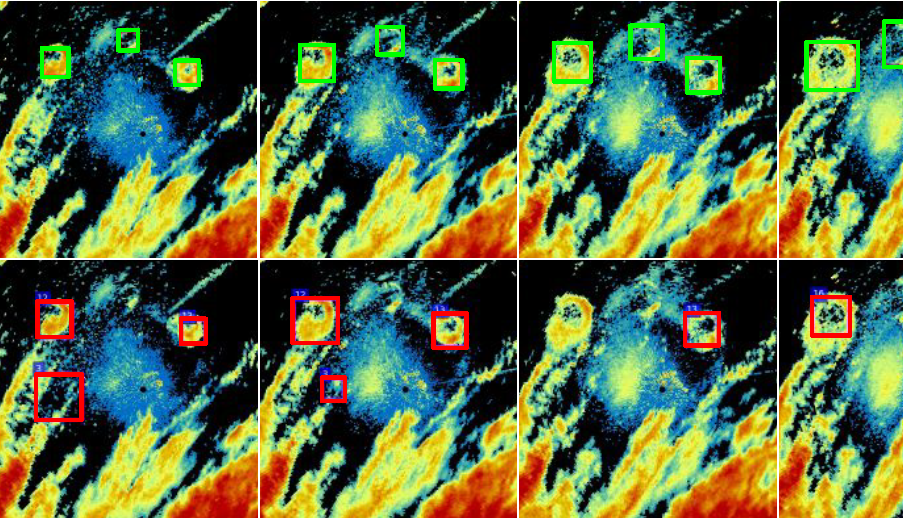
AI FOR SUSTAINABILITY—Deep learning of nanoporous materials for energy-efficient chemical separationsWe present ZeoNet, a representation learning framework using convolutional neural networks and 3D volumetric representations for predicting adsorption in zeolitesGithub code repository · paper · press release |

AI FOR ASTRONOMY—Star cluster classification from nearby galaxiesIn this work we focus on an ecosystem of AI tools for cataloging bright sources within nearby galaxies, and use them to analyze young star clusters.Github code repository · paper |
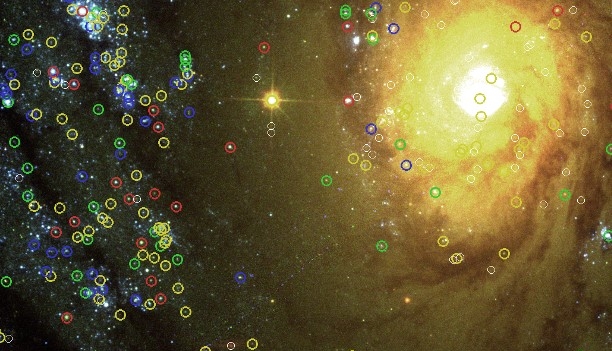
MISC
I'm also a photography enthusiast. → Some of my photos .